In February 2025, a new study from Stanford made the headline “Physicians make better decisions with the help of AI chatbots.”
What was once considered a flashy add-on is now becoming a serious and functional part of healthcare business operations. The following study conducted by the research team details that in clinical decision-making, the chatbot outperformed doctors using conventional methods and was on par with those using ChatGPT. Similarly, in diagnostic testing, an AI chatbot outperformed doctors and AI-powered physicians in a clinical trial.
Building on this momentum, new tools, like Atropos Health’s ChatRWD (a chat-based real-world data analyst), are gaining attention and can generate publication-grade medical evidence from massive patient datasets in minutes. These advancements demonstrate that the future of chatbots in healthcare lies in intelligent exploration. In the U.S., where provider shortages and patient demand are acute, AI co-pilots promise to extend care capacity.
In this article we will explore the business drivers, use cases, and future trends for healthcare chatbots – and why now is the time for health systems to invest thoughtfully in this technology.
Key Business Drivers
Workforce shortages
The U.S. healthcare system is experiencing a shortage of human health resources. The Health Resources and Services Administration (HRSA) projects that by 2026, the total number of physicians will be sufficient to meet only 90% of the estimated demand, a figure that is expected to decline to 87% by 2036.
HRSA further estimates that by 2036, the United States will face a shortage of 139,940 full-time equivalent (FTE) physicians across all specialties. Since healthcare providers are often stretched thin, they are always on the lookout for AI based solutions, such as chatbots.
AI-powered chatbots can assist in alleviating staffing shortages by automating administrative tasks and low-risk clinical duties. This allows human clinicians to focus on providing higher-value care.
Mental health crisis
The U.S. is in the midst of a severe mental health crisis. In 2023 about 1-in-5 U.S. adults reported symptoms of anxiety or depression, and two-thirds of high-schoolers felt persistently sad or hopeless. Yet providers are scarce: an estimated 122 million Americans live in mental-health shortage areas.
Conversational agents or AI agents can help bridge this gap by providing on-demand support, basic counseling, and triage (with privacy and anonymity), mitigating barriers to care.
Rising patient expectations
Digital-savvy patients now expect convenient, 24/7 access to health information and services. Research shows most consumers prefer mobile/web channels for routine tasks, and many are willing to use AI for scheduling or basic triage if it’s accurate and secure. This approach, used at Weill Cornell Medicine, led to a 47% increase in appointments books digitally via an AI chatbot.
Meeting patient expectations for seamless, personalized engagement (via portals, apps or voice assistants) is a competitive and satisfaction driver for providers.
Regulatory and policy pressures
Policymakers are emphasizing digital health and interoperability. CMS and ONC rules reward patient engagement, information access and population health outcomes. Telehealth expansions and evolving HIPAA/QRDA guidelines mean providers must securely track patient interactions across channels.
In this context, chatbots can help organizations comply by improving access (e.g. automated reminders to support chronic-care metrics) and freeing up staff to focus on meeting quality targets. However, looming regulations also mean chatbots must be designed for compliance from the ground up.
Core use cases of healthcare chatbots
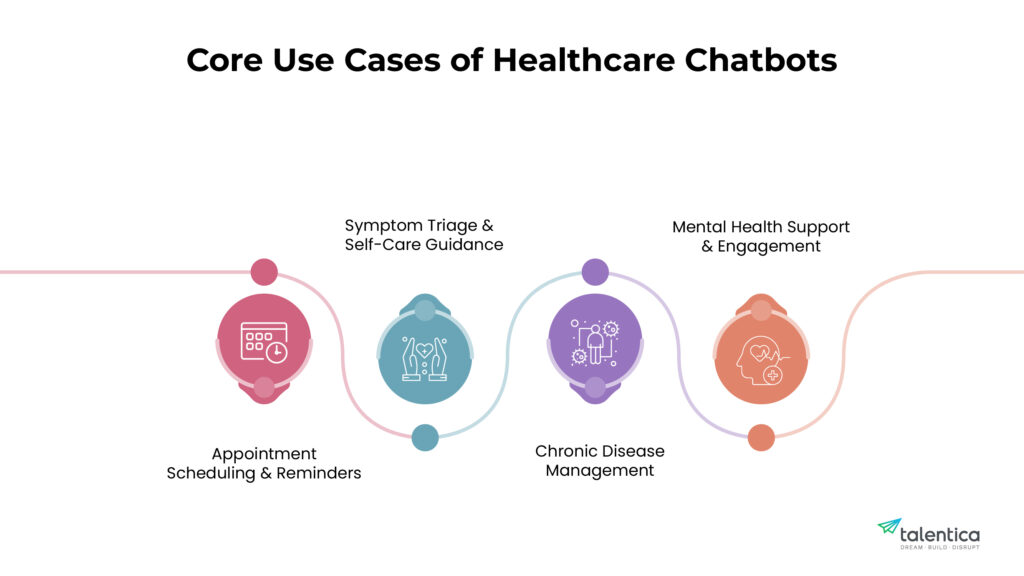
Healthcare chatbots are most valuable in well-scoped scenarios that either relieve routine burden or extend care access. Key examples include:
Appointment scheduling & reminders
One of the simplest, highest-impact uses is automating bookings and follow-ups. AI chatbots can interface with scheduling systems to show availability, book/reschedule visits, and send reminders. In practice this reduces phone traffic and no-shows.
For instance, commercial virtual assistants (like the Ada platform) allow patients to confirm appointments by chat, giving staff breathing room. Automating these tasks not only saves clerical time (and cost) but also improves patient convenience and adherence.
Symptom triage & self-care guidance
Chatbots can serve as first-line triage agents. By asking structured questions, a chatbot collects symptoms and context and can guide the user to an appropriate next step (self-care tips, nurse line, or emergency care).
For example, the Ada Health chatbot interacts with users to assess conditions and recommend actions (advice or escalation) based on AI-driven algorithms. Research on leading symptom-checker bots has shown performance approaching that of clinicians in simulated cases.
While no AI is perfect yet, embedding chatbots can help filter low-risk cases (faster for patients) and flag high-risk symptoms for prompt human review. In COVID-19 and flu seasons alike, symptom bots have already helped triage patient questions at scale.
Chronic disease management
Managing chronic illnesses (diabetes, heart failure, COPD, etc.) requires ongoing support – education, medication reminders, lifestyle coaching. Chatbots can deliver much of this at low cost. Systematic reviews of diabetes chatbots show high patient acceptance and promising outcomes.
For example, chat-based diabetes programs have used gamified lessons, data logging, and periodic check-ins to gradually improve patients’ blood-glucose control. More broadly, AI health coaches can track user data (diet, glucose, weight, steps) and nudge positive behaviors. These bots act as “always-on” care partners, reinforcing clinician guidance between visits and alerting providers when intervention is needed.
Mental health support and engagement
Dedicated mental-health chatbots (e.g. cognitive-behavioral therapy coaches) are an area of rapid growth. Recent meta-analyses find that AI conversational agents can significantly reduce symptoms of depression and distress.
Such bots deliver structured therapy modules (often CBT-based), mood tracking, and just-in-time coping exercises. Because they are private and on-demand, some patients find them easier to use than traditional therapy apps.
In practice, health systems have begun offering these bots as adjuncts to counseling services. While they are not a replacement for human therapists, they can extend the reach of care (for milder symptoms or waitlisted patients) and provide a supportive tool for ongoing mental well-being. As these agents become more sophisticated, they will play an even more integral role in the healthcare industry, providing interactions that closely mimic human conversations.
Trends that are redefining care
The future of chatbots in healthcare is promising. They are evolving fast along several fronts:
EHR Integration
Next-generation bots link into electronic health records and clinical workflows. For example, AI can analyze doctor–patient conversations (voice or text) to auto-fill clinical notes and update records.
Bots integrated with EHR APIs can pre-populate forms, retrieve patient data to personalize responses, and even nudge clinicians about preventive care. This tight integration turns chatbots into informed assistants: they can use a patient’s history (medications, labs, problems) to answer questions and flag anomalies, rather than working in isolation.
LLM-driven personalization
The rise of large language models (LLMs) means chatbots can have more natural, context-aware dialogue. By leveraging patient data (with consent), an LLM-based bot could, for example, tailor medical advice to a user’s health record or past conversations.
Early research envisions “clinic-specific” bots that learn from an institution’s protocols. As these models mature, we expect greater personalization – bots that remember user preferences, adapt content complexity to literacy levels, or switch languages and modalities (text, voice) automatically.
Multimodal and voice interfaces
Healthcare chatbots are becoming multi-modal. Some systems now accept voice input (like Siri/Alexa style assistants for medical queries) or parse images (e.g. patients showing rashes) alongside text. Studies note that adding voice-to-text greatly improves accessibility and engagement.
For patients with low vision, limited typing ability, or those on the move, voice chatbots offer hands-free interaction. In the future, we expect visual interfaces too – for example, augmented-reality-assisted triage or bots that analyze X-rays or photos as part of their assessment.
Conversational AI co-pilots for clinicians
Beyond patient-facing bots, “smart co-pilots” are arriving for providers. These tools monitor EHR streams or lab results and alert clinicians via chat (e.g. “Patient X’s potassium is high – consider rechecking”). They summarize patient histories on-demand (“Doc, the patient’s last 3 visits mentioned fatigue and cough”) and can draft patient letters or order sets.
Such AI assistants can handle routine documentation or info retrieval, letting clinicians focus on decisions. Importantly, this trend reflects a shift: chatbots are not just for patient chat windows, but also embedded in clinicians’ workflow as productivity aids.
Risks and requirements
Deploying chatbots in healthcare demands careful attention to safety, privacy and design:
HIPAA and data privacy
Chatbots handling patient data must fully comply with HIPAA and other privacy laws. Free public models (e.g. ChatGPT) are not HIPAA-compliant by default. Any PHI fed to a bot must be anonymized or secured. Systems must safeguard data at rest and in transit, with audit trails and encryption.
Vendors and providers should sign Business Associate Agreements and ensure bots meet the “confidentiality, integrity and availability” standards that HIPAA requires. In short, privacy cannot be an afterthought: compliance-by-design is mandatory.
Accuracy and clinical validation
Chatbot advice must be accurate and evidence-based. Health AI can hallucinate or regurgitate outdated info if unchecked. Before deployment, chatbots need rigorous testing: validating their outputs against clinical standards and updating them with new guidelines. Some errors are obvious (“Florida poison ivy” quandary), others subtle (“mixing up similar drug names”), so human oversight is crucial.
In practice, content often must be “templated” or curated by experts, not entirely freeform. In high-risk use cases (like triage), conservative defaults and disclaimers are needed. Regulatory frameworks (FDA guidance on SaMD/AI) are evolving, so organizations should stay aligned with emerging rules for AI tools in medicine.
Patient trust and user experience
Even with safeguards, patients may distrust a digital agent. Surveys show 60% of Americans would feel uncomfortable if their provider relied on AI for their care. Trust must be earned by transparency (making clear it’s a bot), reliability (consistent correct answers), and user-friendly design (clear language, empathy).
Simple conversational tone and the option to connect to a human escalates trust. Collecting user feedback and monitoring chatbot performance (e.g. satisfaction ratings, resolution rates) is vital.
Hybrid human–AI collaboration
A key lesson from recent studies is that the best outcomes come from combining humans and bots. Doctors aided by chatbots can diagnose as well as the bot alone, whereas doctors with only search tools were slower. This suggests chatbots should augment, not replace, clinicians.
Workflow design should incorporate handoffs: e.g., a chatbot handles initial screening, then flags urgent cases for nurse review. Clinicians must be trained on how to interpret and use chatbot outputs. In short, success requires defining clear boundaries: what the bot does, what the human does, and how they communicate.
Why now and keys to success
The convergence of capable AI and urgent healthcare needs makes this an ideal moment to invest in chatbots. Advances in LLMs, cost-effective cloud deployment, and FDA/ONC interest align with sprawling patient demand and strained staff.
Several recent trends reinforce this: hospitals saved millions by automating scheduling and call handling, digital therapeutics gained traction, and mainstream AI adoption rose sharply in the past 3 years.
Organizations that wait risk falling behind in efficiency and patient experience. To succeed, health systems should outsource or build bots thoughtfully. Key success factors include:
Strategic scoping
Start with high-impact pilot use cases (e.g. post-discharge follow-up calls) rather than vague “AI” hype. Define clear goals and metrics (time saved, engagement rates, outcome improvements). Involve clinicians and patients early to ensure the bot meets real needs.
Compliance-first design
Bake privacy and security into the architecture from day one. Work with IT and legal teams to ensure data handling meets HIPAA. Document the chatbot’s knowledge sources and limitations as part of risk management.
Seamless integration & usability
A chatbot must feel like part of the care journey. Integrate it with EHRs and patient portals so it’s accessible in existing apps. Design the conversation flows to match clinical workflows (for clinicians) and health literacy (for patients).
Include easy fallback options (e.g. “talk to a nurse” buttons) and support channels. Focus relentlessly on user experience – a high-tech tool is useless if patients find it frustrating.
Ongoing oversight and improvement
Once live, monitor the chatbot’s performance (accuracy, patient satisfaction, safety reports). Regularly retrain the AI on new medical guidelines and feedback. Update scope as needs change (e.g., add new languages or care lines). Cultivate a multidisciplinary team (tech, clinicians, compliance) to refine the bot over time.
Conclusion
In sum, the future of chatbots in healthcare lies in AI advancements. The evidence and technology are here: rigorous studies and real-world pilots show they can be safe and effective. As per Data bridge market research report the global healthcare chatbots market is expected to account for USD 1179.81 million by 2030.
For providers looking to reduce costs, improve access, and meet patient expectations, now is the right time to go for chatbot solutions – provided they proceed with careful planning, strong clinical oversight, and a focus on trust and usability.