Artificial Intelligence (AI) is the axis of the 4th Industrial Revolution. It has revolutionized business operations, and there is hardly a sector left that hasn’t experienced its groundbreaking impacts. Modern societies and our daily lives are also getting shaped by it.
AI’s ability to automate repetitive learning and analyze data simplifies adding intelligence to existing products. Its tools like automation, conversational platforms, bots, and smart machines, fused with actionable data insights, transform other technologies too.
According to the 2024 PwC’s Global Artificial Intelligence Study, AI could contribute up to $15.7 trillion to the global economy in 2030. This highlights its significant impact on economic growth and innovation across various sectors.
To understand the impact of AI, let’s dive deep into the use cases of AI across various industries.
Use cases of AI
AI in Healthcare:
The sector has grown exponentially in recent years. But AI is set to transform it further with its unique capability to generate value from the databases of billions of patients.
Some of the most promising use cases are mentioned below –
1. Real-time patient care:
- Chatbots with Natural Language Processing (NLP) can reduce patients’ dependency on physical visits to a doctor.
- In telemedicine, AI can help doctors automate diagnoses and deliver prescriptions based on patients’ symptoms, past diagnoses, prescription data, family medical history, etc.
- AI can make real-time patient monitoring easy, accurate, and reliable. AI-powered health devices can provide real-time vitals, blood sugar levels, etc., using onboard sensors and proactively alert doctors.
Read also: How healthcare can benefit from AI?
2. Early detection of diseases:
- AI can quickly process the medical imaging and diagnosis data to give near exact cause of an illness or injury and confirm a diagnosis.
- Researchers in Boston claim that in the near future, AI would significantly advance lung cancer screening by detecting early signs of the disease years before doctors would discover them on a CT scan.
- To identify tumors in their earliest stages, several AI technologies have been developed. Scientists from the Massachusetts Institute of Technology and the Mass General Cancer Center in Cambridge created the new AI tool known as Sybil. According to one research, it may properly predict a person’s risk of developing lung cancer in the coming year 86% to 94% of the time.
- Similarly, a new study published in Frontiers shows the potential of AI for early detection of congenital heart disease in newborns. These AI tools could significantly improve diagnostic accuracy and efficiency, freeing up healthcare professionals for other vital tasks. (11 Jan 2024)
3. Assisted surgery:
- AI, combined with computer vision, can increase precision during surgery.
- Intelligent robot-assisted surgeries can prevent human error due to exhaustion and fatigue.
4. Drug discovery:
AI speeds up the development of drugs and vaccines, which makes it feasible to find potential treatments and cures more quickly and efficiently. For Examples:
- The Oxford-based biotech startup Etcembly just created medical history by using generative AI to create an immunotherapy drug. (Sep 20, 2023)
- Insilico Medicine, a Hong Kong and New York City-based AI-driven biotech startup, has announced that their new generative AI-designed medication for COVID-19 (Effective against all variations) has begun Phase I clinical trials. Once accepted, the oral medicine would offer “a promising alternative” to currently available antiviral treatments. (September 5, 2023)
- Recently, researchers have achieved success using a hybrid quantum generative AI model to create promising new cancer drug candidates. This breakthrough suggests a bright future for this technology in accelerating drug discovery, using the capabilities of both classical and quantum computing. (February 20, 2024)
AI in Fintech:
AI has transformed the fintech industry by making digital transactions and data aggregation a new way of life. Its solutions are aimed towards meeting the critical needs of the financial sector.
Let’s check out some of the popular use cases of AI in fintech –
1. Assisted decision-making:
- AI’s assistance in data-driven decisions can reduce management costs and improve decision quality.
- It can improve the accuracy of predicting the risk of disbursing loans to prospects and, for insurance, lower the probability of damages to the insured and insurer.
- AI can review parameters like risk tolerance, life expectancy, market situation, etc., to help make informed decisions for asset management and investment management.
2. Automated support:
- AI-integrated finance can empower chatbots to deliver human-like expert advice and customer support at a much lower cost.
- AI can assist insurance companies in automating the underwriting process to speed-up operations. It can also help with raw information analysis to improve customer-related decisions.
3. Fraud detection and claim management:
- AI’s analytical tools can collect pieces of evidence and analyze the data required for conviction purposes.
- AI tools can understand and monitor the behavioral patterns of a user to identify and warn about possible fraudulent activities.
- Insurers can leverage AI’s ability to handle a vast range of data in a short time, reduce overall processing time, and curb costs to improve the customer experience.
- A real-world example of GenAI use case in banking comes from Bank of America. The bank’s AI system analyzes billions of daily transactions to detect fraudulent credit card activity. The system identifies red flags like unusually large purchases or transactions made from atypical locations.
One of our fintech clients, Citrus Pay, improved the payment system with AI implementation. Click here to read.
AI in Real Estate:
AI’s impact has proliferated the real estate sector on a granular scale. It now covers from helping agents with lead generation to transforming the search process of homes.
Here are some of the prominent use cases of AI in real estate –
1. Home search for clients:
- Businesses can leverage AI for a better customer experience. Its ability to read patterns and simplify the search process can improve the conversion ratio of prospects to clients.
- AI-integrated applications can serve as conversational interfaces to answer customer queries.
2. Lead identification for agents:
- AI can assist agents in identifying ideal clients by analyzing data points that differentiate serious buyers from non-serious ones.
- Natural Language Processing (NLP) can find contacts who are more engaged with agents and help with resolving queries.
HomeUnion built a feature-rich product with data-driven insights that help home buyers to make data driven investment decisions using AI. Click here to read more.
AI in Marketing & Advertisement:
AI has completely transformed the online marketing and advertising landscape. Advertising platforms leverage AI to auction and place ads in real-time.
Let’s take a look at various use cases of AI in marketing & advertisement –
1. Budget optimization & targeting:
- AI tools can automatically optimize advertising spending and target while processing the same.
- Understanding what can drive better outcomes is easier with AI tools reviewing results to learn actions like spending changes, targeting changes, etc.
2. Ad creation and management:
- AI can conduct tactical data analysis quickly and reach conclusions based on campaigns and customer behavior to boost ad performance.
- Marketers can integrate AI to focus on strategic initiatives to form their own AI-enabled campaigns. They can also leverage AI for ad copy creation and variations.
- Marketers can save time using AI systems in ad campaign management and analysis.
3. Improved customer relations & real-time personalization:
- AI can help deliver the right personalized messages to customers at the correct time.
- Marketers can leverage AI for better real-time analytics and faster decisions. They can then identify risks for customers and target them with enriched information to increase brand engagement.
AI implementation helped Opera Mediaworks manage 8 billion requests per day. Click here to read.
Key AI technologies in demand
Implementing AI technologies depends on business needs, technical capacity, product and service, and others.
1. Generative AI:
Generative AI is a type of artificial intelligence that can generate several kinds of content, such as text, videos, code, images, audio and stimulations. In order to create fresh and unique content, generative AI models use neural networks to recognize the patterns and structures within existing data.
Generative AI applications:
- Generating pictures based on a scene, subject, style, or place; converting semantic images into photos; transforming one image into another; creating 3D shapes; or enhancing image resolution.
- Generation of text-to-speech, musical content for advertisements/marketing campaigns, and speech-to-speech conversion.
- In the marketing, gaming, and communication sectors, generative AI is frequently utilized to generate conversations, headlines, or advertisements. It is also used to create customised content like social media postings, blog articles, product suggestions.
- In applications that use code, such as those that generate, complete, review, fix bugs, refactor, and check for style.
- The generation of natural language responses to user input, data visualization, creation of synthetic data, and the resolution of mathematical issues are some other generative AI applications.
2. Machine learning:
You can use the data to automate the analytical model building with machine learning. So you don’t need to program it to generate accurate predictions explicitly. ML systems can learn from data, identify patterns, and make decisions with minimum human intervention.
But a strong data pipeline is a must for ML models to iteratively improve prediction accuracy.
ML applications:
- Facilitate automatic decision-making with minimum human intervention, e.g., price prediction, self-driving cars, etc.
- Suitable for a task to identify trends in customer behavior and business operation patterns.
- Simplify product recommendations for e-commerce websites by tracking customers’ behavior based on previous purchases, searching patterns, and cart history.
- Suitable for cataloging and detecting an object in a digital image. ML’s image recognition technique analyzes data based on pattern recognition, face detection, or face recognition to deliver results.
- Read and compare millions of online transactions to differentiate the legitimate from the illegitimate and ease the tracking of monetary fraud.
3. Computer Vision:
It is a field of artificial intelligence that helps computers interpret the visual world. It uses deep learning models to process images and videos to help machines identify and classify objects to perform valuable tasks.
Computer vision applications:
- Helpful in building face recognition systems.
- Find defects/damages in the product during the manufacturing process.
- Improve automated checkouts and product identification in automated retail stores.
- Useful transportation for self-driving cars, pedestrian detection, parking occupancy detection, traffic flow analysis, and road conditioning monitoring.
- Prevalent in healthcare for X-Ray analysis, CT and MRI, cancer detection, blood loss measurement, digital pathology, and movement analysis.
4. Natural Language Processing (NLP):
AI’s branch gives computers the ability to understand text and spoken words like a human being in real-time. It combines computational linguistics with rule-based modeling of human language and statistical ML and deep learning models.
NLP applications:
- Important for virtual assistants/chatbots that can respond to human queries without involving any human. Serve well where there is heavy traffic.
- Useful for language translation tools to translate voice into different languages.
- Enhance text analytics to convert unstructured data into meaningful data suitable for analysis.
- Performs well in social media monitoring where unstructured data analysis such as customers’ likes and dislikes is required.
- Improve hiring and recruitment processes. Recruiters can use the information extraction technique with named entity recognition to get information like skills, name, location, and education.
- With NLP’s text classification techniques, emails can be filtered into different categories.
5. Deep learning:
It is a subset of AI inspired by the human brain’s neural network’s functioning and imitates how a human brain learns. It is not bound by strict indications responsible for determining the correct and incorrect. The system can draw its conclusions, and the basic parameters are set with deep learning related to the data. It trains the computer to understand pattern recognition based on various processing layers.
Deep learning applications:
- Useful to create systems that can automate human tasks like self-driving cars, and virtual AI assistants that can be customized as the user.
- Can detect fraud in insurance claims.
- Improve customer relationship management by noting why customers buy, when they buy, and what keeps them around.
- Churn out and process the large volume of data to identify patterns and insight purposes.
- Valid for the entertainment industry to aggregate volumes of data based on viewers’ watch lists and then create a personalized experience.
- Can make the manufacturing sector more efficient to eliminate any production-related errors.
Successful AI implementation has some prerequisites
Business implementation of AI faces various challenges. Therefore, knowing the parameters and conditions before implementing AI can change the outcome to a large extent. Also, you can check our blog on top considerations for implementing Machine Learning in fast-growing tech companies for a detailed explanation.
1. Labeled data:
Labeling a massive amount of data is a critical process used to set the context before leveraging it for model training. Before you start the implementation process, ask the data-driven questions given below.
- Do you have a data-driven culture?
- Do you need labeled data, and if that’s the case, do you have it?
- Do you have a reasonable quantity of data to implement AI/ML?
2. Data pipeline:
Involves a series of steps that helps in moving the data generated from a source to a specific destination. Having a robust data pipeline ensures data combining from all the disparate sources at a commonplace, and it enables quick data analysis for business insights. Further, it provides consistent data quality. Ask the data pipeline-related questions given below for more clarity.
- Do you have an AI/ML data pipeline right from model generation to validation?
- If you have no data or limited data, do you have a well-tested data generation strategy and domain experts for doing so?
3. Right model:
Choosing the wrong AI model will jeopardize your entire AI implementation. A large amount of data with the wrong choice of AI model could lead to huge training data compared to traditional data, thus, obstructing the AI project. To choose a suitable model, consider answering the questions given below first.
- Do you have a dedicated AI/ML team that can iteratively improve the AI/ML model?
- Do you have data scientists who can develop AI/ML models while keeping an eye on engineering aspects?
- Do you have the resources and time to invest in AI/ML model research?
- Do you have the required infrastructure to serve AI/ML models?
We have created an AI implementation checklist for fast-growing tech companies based on our experience working on AI solutions for Startups across various industries. Check it here: AI/ML implementation Checklist for Startups.
A step-by-step AI Implementation Strategy
AI’s upcoming impact on the global economy may make you think of leveraging the technology right away. If your organization doesn’t have AI-based solutions as of now, do not rush into it. The best option is to plan AI implementation in your business operations first. Before that, you should have a reasonable understanding of where to implement it and how you can go ahead with it in your business. If you do so, the method will give you a better understanding of the right technology and then help you with automating and streamlining the process.
Let’s check out the steps.
1. Data Acquisition:
Gathering customers’ data is essential to understanding behavioral patterns. It creates scope for a strategy that increases customer traffic to your product.
- Data collection: It is a process that involves gathering and measuring information from multiple sources. Collect the data to develop AI and ML solutions, then store it specifically to solve business problems.
- Data pipeline: Create a data pipeline that the engineers use to collect, transport, process, transform, store, retrieve, and effectively present the data. All these steps are essential in achieving the result of a data-driven application.
- Data validation, clean up: In this step, ensure the quality of input data before using it to develop models and insights. Data validation includes data checking for the right type, the range of values, data validity, and consistency.
- Data exploration, analysis: It is also called Exploratory Data Analysis (EDA). It is a process where the data is explored and understood with the help of statistical and visualization methods. You can finalize the model or algorithm for the upcoming steps based on the patterns revealed.
2. Model Development:
Developing an AI model is considered one of the longest stages in the development process. The purpose is to achieve a high degree of accuracy here. Three essential components determine the accuracy of AI models-
- Feature engineering: In this step, transform the raw data into features to present the underlying problem to the predictive models. It further enhances the model accuracy on unseen data. Here the inputs are turned into things that the algorithm can understand.
- Model training: After you feed the data into the computer system, the movement offers a specific prediction with each cycle. The parameters can be adjusted according to the need.
- Model evaluation: At this stage, engineers should assess the correctness of models chosen in the previous steps on the test data. These test data have the data points identified by the model before.
3. Deployment:
Here, integrate an AI model into an existing production environment. The aim is to make business decisions data-drive, better, and more effective.
- Product integration: Integrate the product in this step to drive better value from data and streamline processes and operations. The step also identifies specific parts of data and their integration through API-led AI.
- Validation: Validating the algorithms by themselves means that it solves the problem given to them. Use a common strategy of embedding the AI in an artificial environment responsible for simulating data it meets.
4. Monitoring:
In this last step, the AI teams across verticals agree that the data and models should be appropriately monitored in production. Assess the impact on the models accurately in this step, be it negative or positive on the business outcomes. The availability of labels helps in calculating and analyzing standard model validation metrics like error/loss functions, precision/recall, etc.
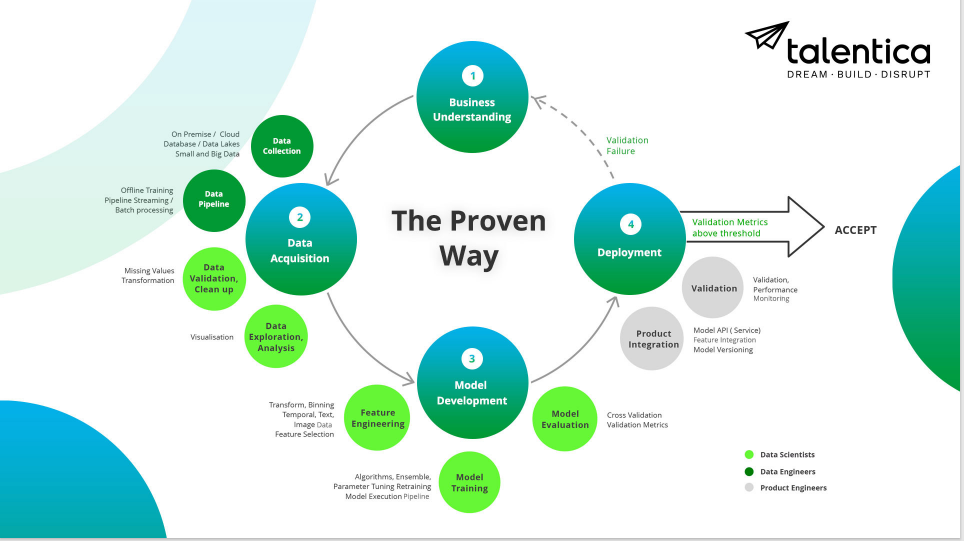
AI/ML Implementation Cycle
Now, moving on to the next stage, check out the tools and platforms that you can leverage to implement AI successfully.
Most common AI implementation mistakes:
It is essential to adopt AI, but it is also equally important to do it rightly. Here are a few common mistakes that businesses need to avoid:
1. Unclear goals and KPIs:
Starting without a clear understanding of the business goals is a sure-shot way of getting confused along the AI adoption process. Having defined KPIs that you can measure and clear, measurable, and achievable goals is necessary to define the project’s scope and calculate its impact on the business.
Here are a few questions that you must ask before starting:
- What real-life challenge are you trying to solve?
- Have you foreseen how the end product will look post-AI implementation?
- How long will it take to get ROI?
- What is the expected cost of the project?
2. Starting late:
A matured architecture may not be well suited to AI. This may lead to spending a good amount of resources to manage arising tech issues during implementation. The AI algorithms built on such architecture may result in substandard results or complete failures.
On the other hand, you can build AI algorithms easier, cheaper, and faster if you start early. It is much easier to plan and add AI capabilities to future product feature rollouts.
3. Isolated POCs:
Another common mistake is to focus on standalone proofs-of-concept. These POCs work perfectly in a stable test environment where the data is controlled but can fail in a natural production environment where the information is unpredictable.
So focus should be on production-ready POCs. A quick POC that doesn’t last more than two months would be worth the trial to bring confidence. It is advisable not to be aggressive at this stage, as AI problems take a toll on parameter tuning, resource optimization, and performance.
4. Insufficient data:
There is a strong correlation between the success of the AI implementation and the quantity of quality data pipeline used for model training and improvement. Without a proper data pipeline, AI models cannot generate accurate results.
A data generation mechanism is required to build a healthy pipeline if a proper data pipeline is unavailable.
5. Insufficient skills:
AI implementation requires considerable investment in the team. The team typically consists of data engineers, data scientists & domain experts to build good mathematical algorithms. These algorithms are translated into software solutions by product development teams.
Lack of experience or absence of the right talent in the team is one of the most common reasons for AI implementation failures, and it is also the major cost factor in the entire project budget.
It is advisable to have a team where data scientists can easily connect with the product engineering teams to avoid confusion in interpreting mathematical algorithms and speed up the implementation process.
Tools & Platforms used in AI implementation
Here, we listed down some of the primary tools and frameworks you can leverage to implement AI in your business.
- Scikit Learn
- TensorFlow
- Theano
- Caffe
- MxNet
- Keras
- PyTorch
- CNTK
- Auto ML
- OpenNN
- H20: Open Source AI Platform
- Google ML Kit
Final Words
AI is transforming almost all sectors, and various fast-growing tech companies and enterprises are implementing it to power their products and services with intelligent computational power of AI. This article has tried to explain multiple use cases of implementing AI across industries. We also discussed the use cases of implementing different AI technologies like Generative AI, Machine Learning, NLP, Deep Learning, and Computer Vision.
Multiple perquisites impact the success of AI implementation, primarily the availability of labeled data, a good data pipeline, a good selection of models & the right talent to build the AI solution finally. Once these perquisites are met, a step-by-step process can be followed to create effective AI models accurately.
We hope that the blog simplifies your AI/ML implementation journey.
Need AI development services? We have developed AI solutions that are impacting millions on daily basis. We can help you with AI development teams consisting of AI experts, Data scientists, developers, UI/UX experts, DevOps experts, etc. who have worked on over 30+ challenging AI implementations. You can reach us by clicking here.